Blog
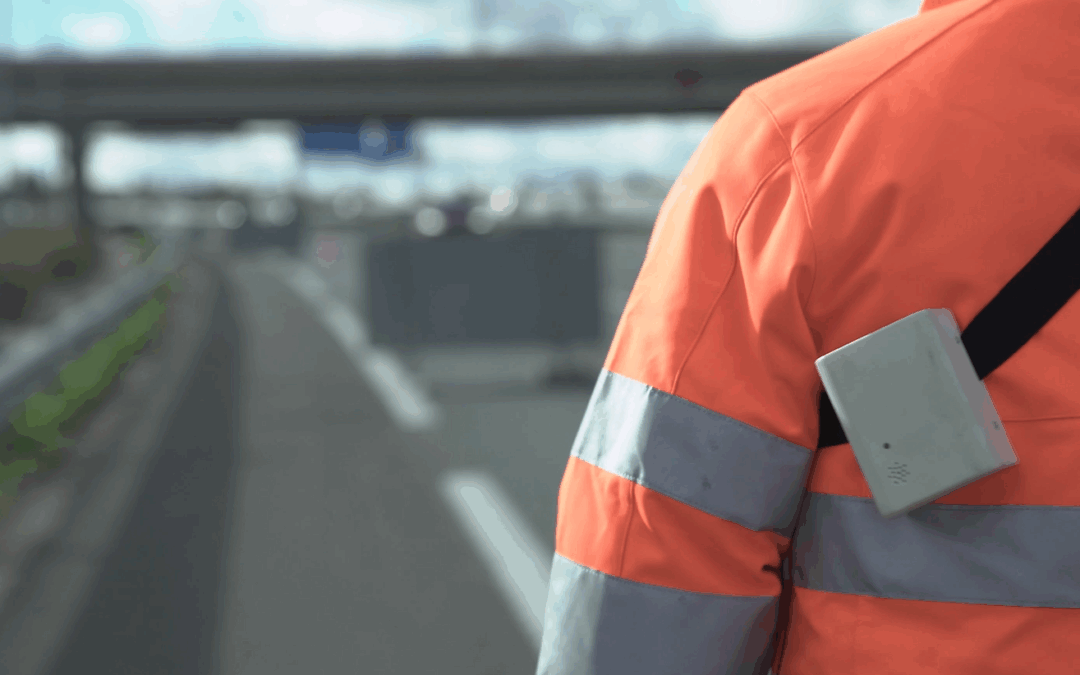
Innovating with intelligence: How we apply AI to our projects
In an increasingly interconnected and technology-dependent world, the use of artificial intelligence (AI) has become a fundamental pillar for improving the efficiency and effectiveness of operations across various industries. At Globalvia, we strive to advance daily...
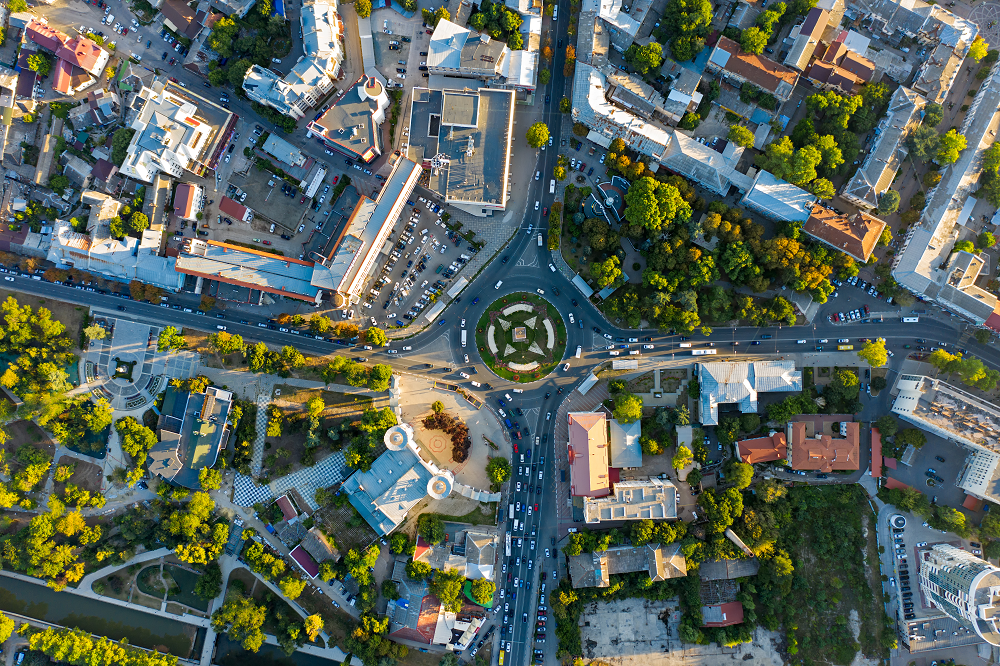
Mobility and economy: the binomial that defines the development of cities and countries
Mobility is a fundamental pillar of economic development. How can a city grow without an efficient transportation system? Without adequate infrastructure, cities and countries see their capacity for growth limited, affecting both business competitiveness and the...
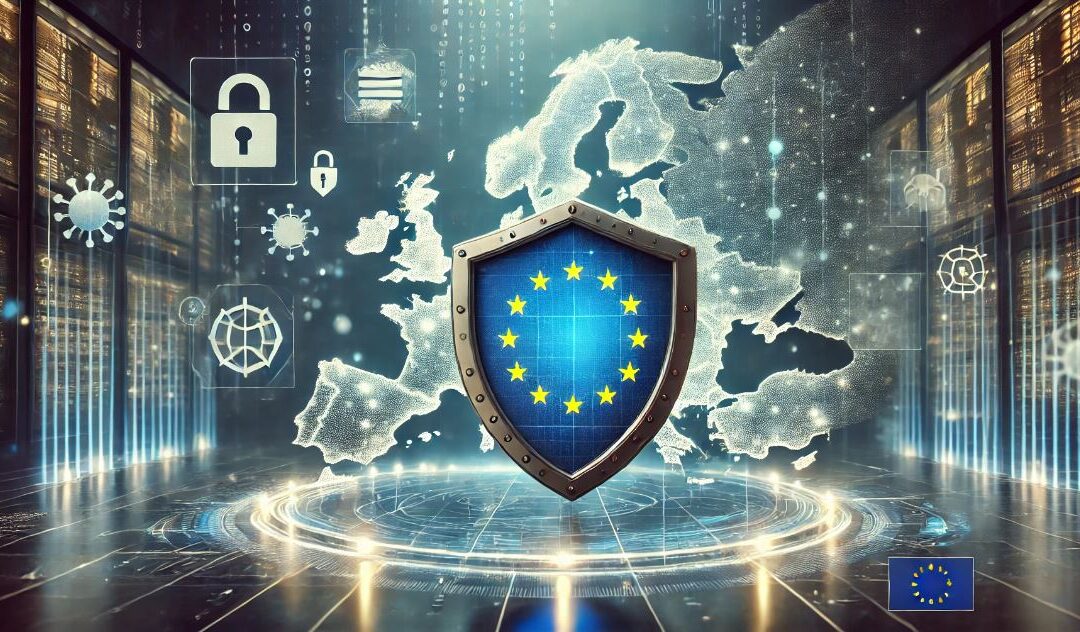
NIS2: The European directive that promises to reduce cyberattacks and strengthen digital resilience in the EU
The arrival of autumn is accompanied by the transposition of the long-awaited NIS2. This European regulation called the NIS2 Directive, for its acronym in English, Network and Information Security 2, is an expansion of the scope of its predecessor, the original NIS,...
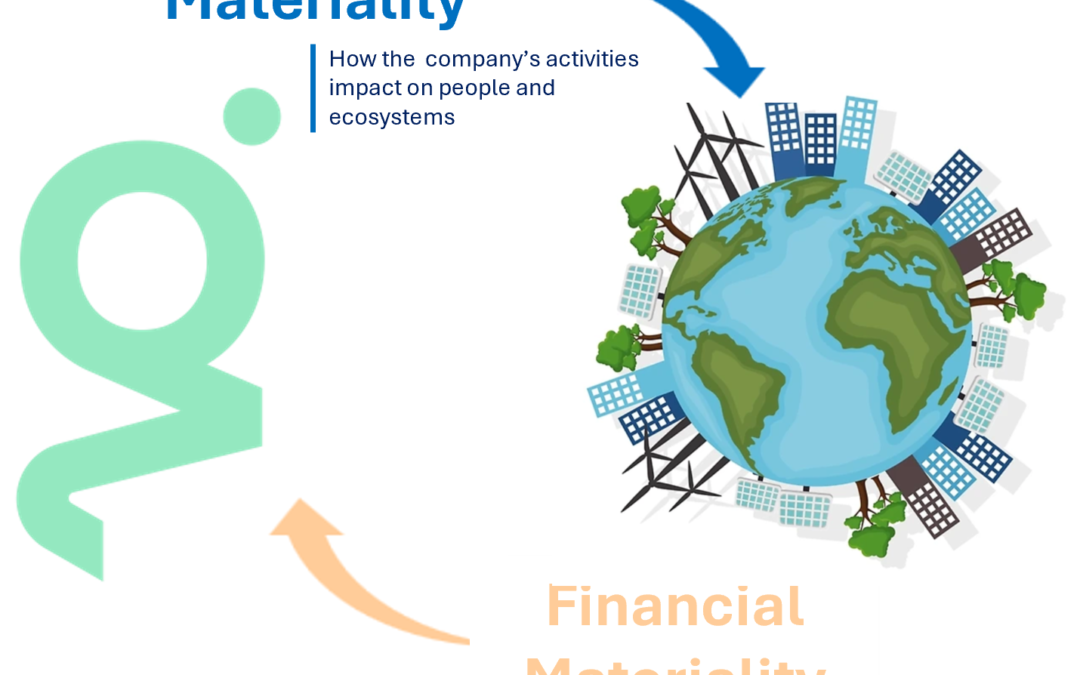
Double Materiality: Our 360º ESG Impacts
Double materiality is a concept according to which companies must consider and analyze how their actions impact people and the environment -Impact Materiality-, but also how sustainability and climate change aspects can affect their financial performance -Financial...
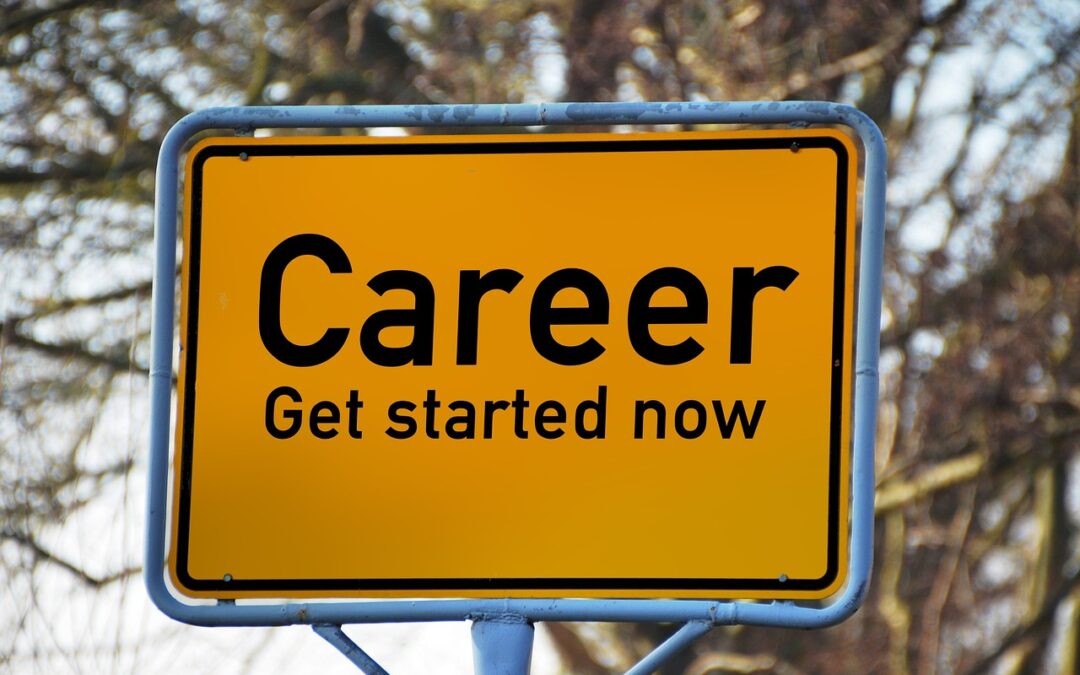
Management careers: myth versus reality
One of the most common questions that Talent teams receive, both from people who are part of the organization and from candidates, is: "what is my career plan?" On July 3rd, the seminar "Management Careers: The Myth Against Reality" was held, organized by LHH. The...
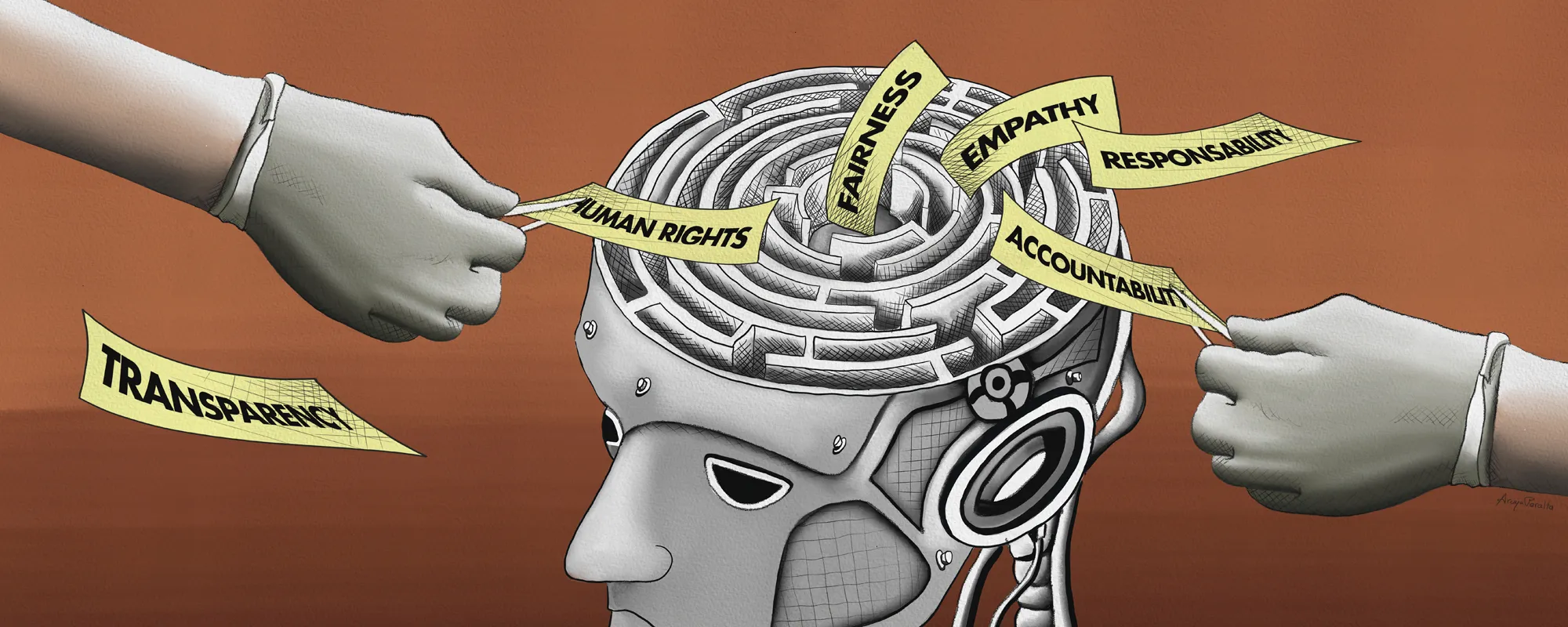
Artificial intelligence and ethical culture
Business organizations that seek sustainability, that is, to endure over time, are following two paths or, perhaps, the same path in two directions. If we were to place ourselves on the Earth's surface, we would say that, on the one hand, they are training astronauts...
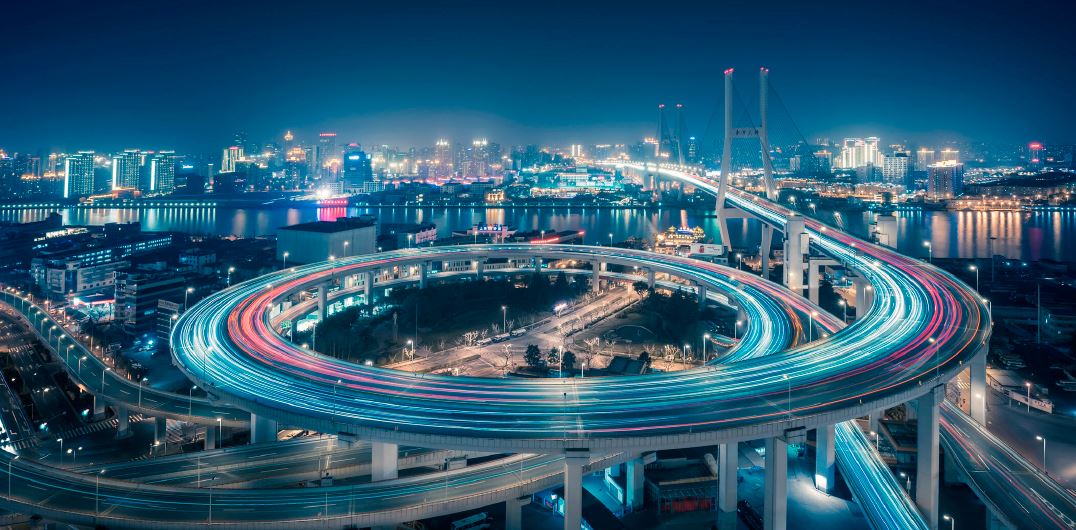
Greenfields, Brownfields… and now Bluefields, the new PPPs for digital roads are coming
After the pandemic, the infrastructure sector is focusing on new challenges to face again potential disruptions in the future: intensifying its operational resilience, the affordability of infrastructure, the need for sustainability and a critical enabler, the...
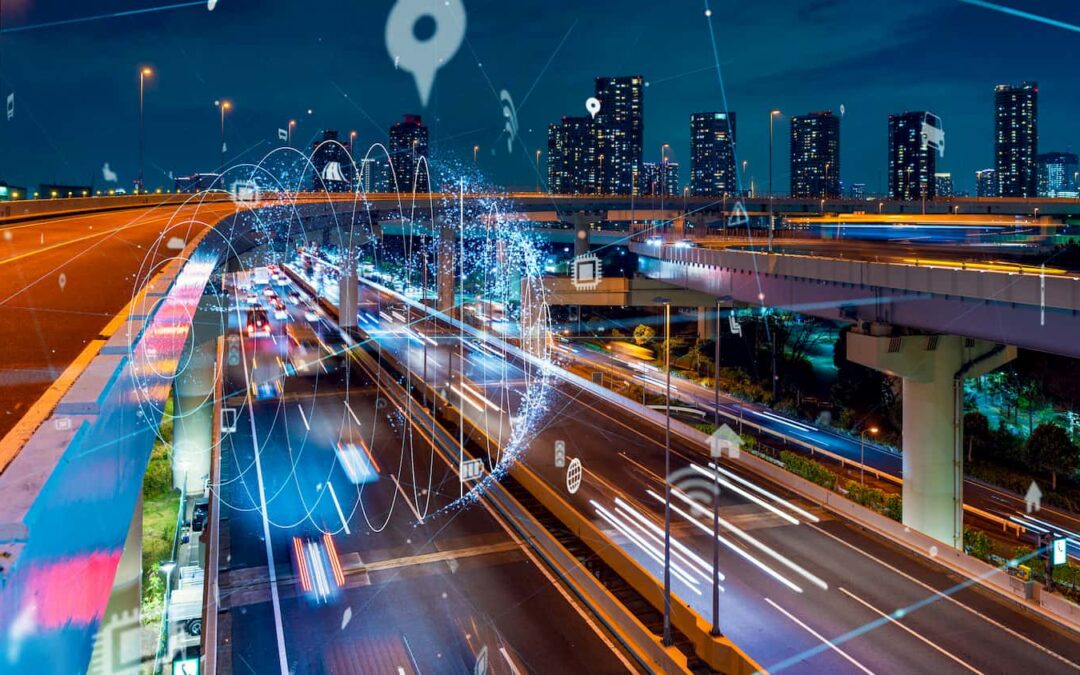
Technology as a differential value in Globalvia’s Internal Audit
The transformation in how we move and the continuous change in the infrastructure where we do it is a reality. Technology has permeated all facets of life, including internal audits. In the complex corporate ecosystem, similar to a vast metropolis, transportation...
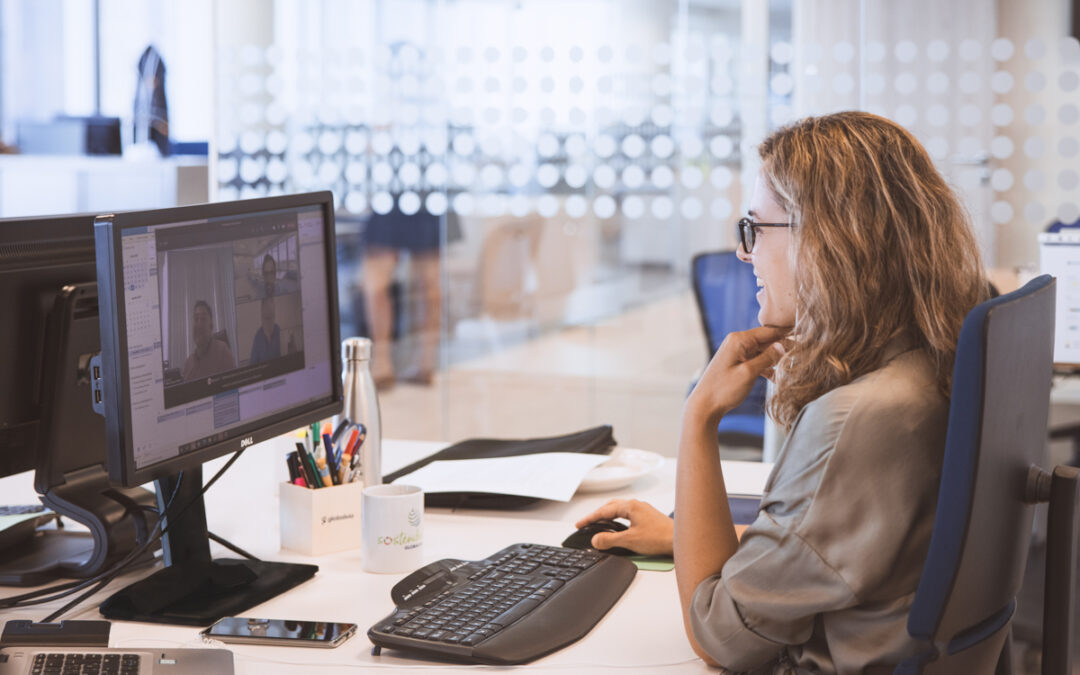
A glimpse into the Compliance function at the companies
By now, we are all familiar with the term “Compliance,” and we are aware of its importance for companies. We know that having a culture of compliance accredited through a “Compliance Model” can constitute an exemption or mitigation of the company's criminal liability,...
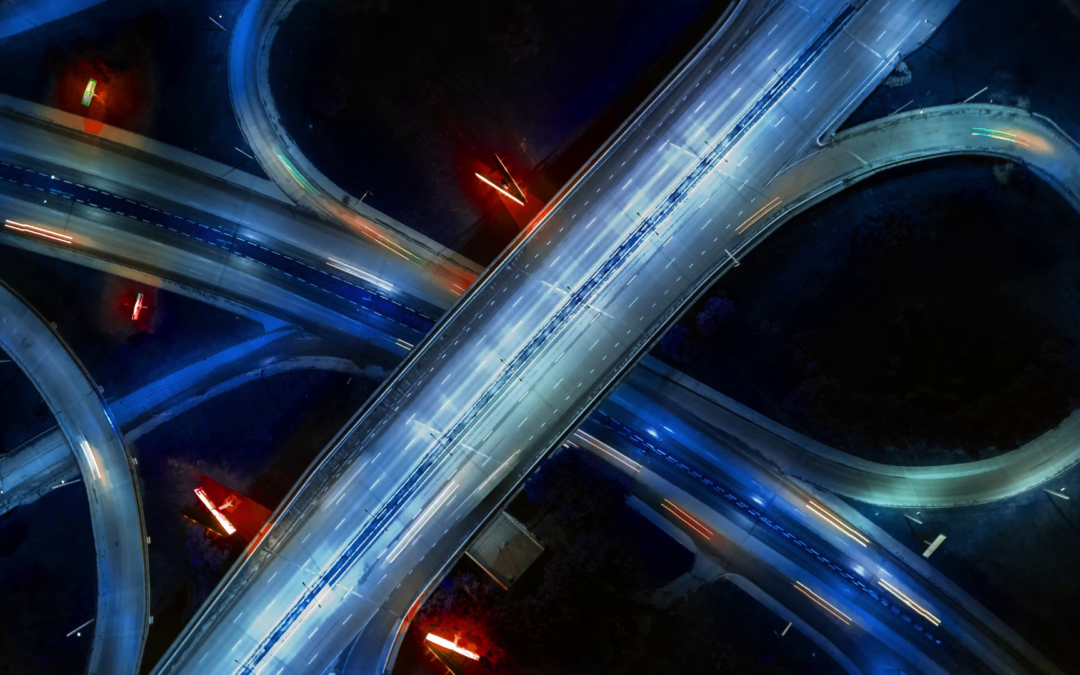
The strategic value of technical management of assets
In the constantly evolving world of transport and mobility infrastructure, technical management has emerged as a critical component for the success of companies operating in this sector. Whether this involves the operation and maintenance of highways, bridges,...
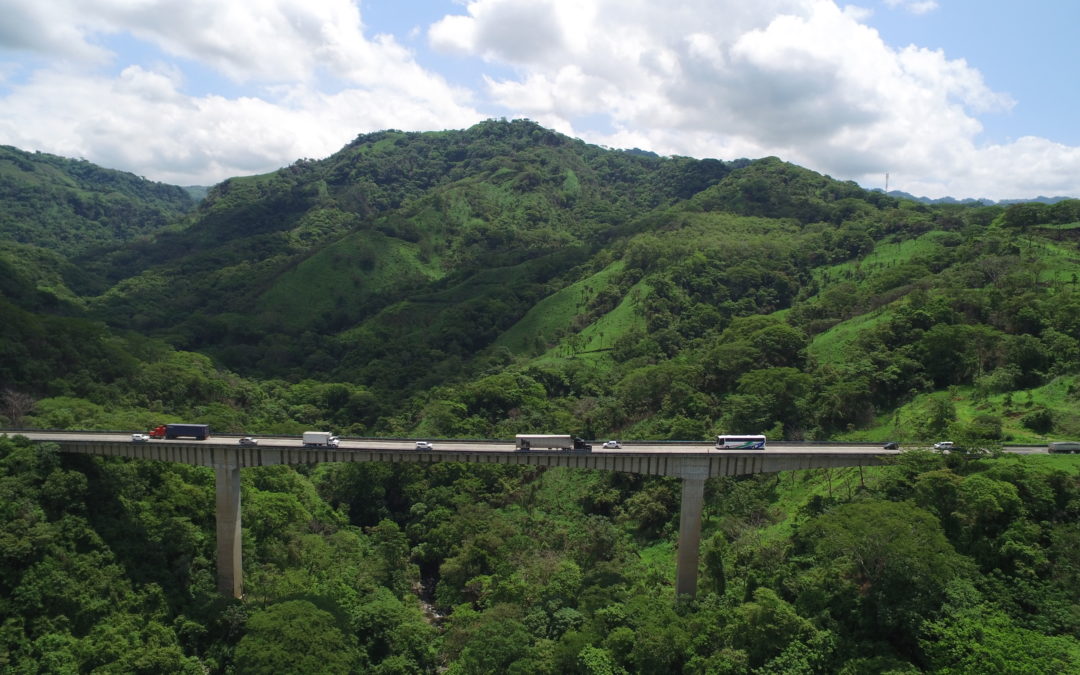
Innovation through green-tinted spectacles
Sustainability is one of Globalvia’s key drivers. It is also one of the most important drivers in the modern world. Thinking of innovation today without factoring in this component means not understanding the reality we live in. Every day we see hundreds of new...